Navigating Gender Preferences to Shape Fairer Online Labor Markets
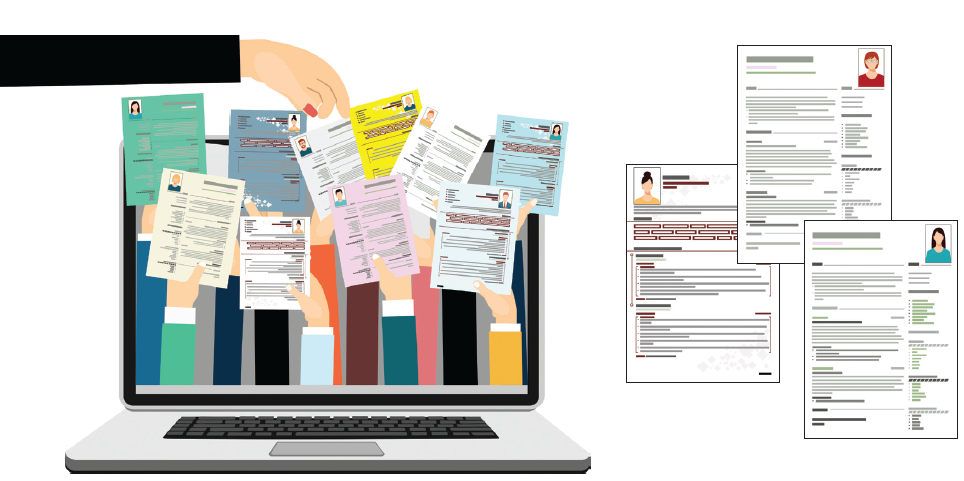
In an era where efficiency and equality are of the essence, cyberspace has become an increasingly popular avenue to perform an array of conventional functions that can benefit from the enhanced cost-effectiveness and removal of geographical barriers. While this can explain why online hiring has become a burgeoning practice, the process can also be susceptible to bias-related issues.
In the first academic study of its kind, Prof Jing Wang and her co-author examined gender hiring bias in the online labor market and found evidence of a preference in favor of female workers, an observation in stark contrast to the discriminatory behaviors against females revealed in most traditional labor market research. In her experiments, she found that females with uncommon names enjoyed a 17% increase in the chances of being hired after posting a profile photo, compared with only 5% for males, which suggests that revealing their gender gives a disproportionate advantage to females.
To find out more about the mechanism underlying the female hiring preference, Prof Wang conducted a further experiment which showed that female-associated traits such as trustworthiness and cooperativeness help overcome the uncertainty and opportunism of online marketplaces. Prof Wang went on to suggest workers, especially male, provide more information to their profiles to signal trustworthiness and cooperativeness, and female workers upload their photos to maximize their gender advantage.
As online labor marketplaces continue to grow in size and reach, Prof Wang believed it is imperative for policymakers to establish rules to promote equality in labor participation and generate socially efficient hiring outcomes.
Prof Jing Wang is Associate Professor of Information Systems, Business Statistics and Operations Management. Having earned a first degree in computer science and doctoral studies in management information systems, Prof Wang has published articles in Proceedings of the National Academy of Sciences (PNAS), Management Science, Information Systems Research, Journal of Management Information Systems, as well as Data Mining and Knowledge Discovery.